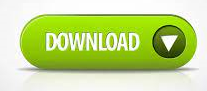


However, incompleteness can disrupt the assessment evaluation, making the system unable to compare incomplete tuples. To analyze complex data, such as performing similarity search or classification tasks, KDD processes require similarity assessment.

However, as activities such as data acquisition, cleaning, preparation, and recording may lead to incompleteness, impairing the KDD processes, specially because most analysis methods do not adequately handle missing data. The continuous growth in data collection requires effective and efficient capabilities to support Knowledge Discovery in Databases (KDD) over large amounts of complex data. FiSmo can be employed for experimentation of computational techniques and systems designed to work with images and videos from emergency situations. Furthermore, the extracted feature vectors are also available, providing features of color and texture. All datasets were preprocessed according to the involved context, including annotation steps carried out by a set of subjects, training images and ROIs. The available data is composed of four image and two video datasets: fire/smoke detection in images fire segmentation in images smoke segmentation in images content-based image retrieval temporal seg-mentation of fire segments in videos and fire detection in videos. These works were focused on the analysis of images and videos regarding the presence of fire, smoke, and explosions in emergency situations.

These datasets were employed in the context of the RESCUER Project they were used in the experimental analysis of techniques created in a set of works carried out at the Databases and Images Group (GBDI) of the University of Sao Paulo. In this work, we present FiSmo, a compilation of datasets from emergency situations, composed of images, videos, regions of interest (ROIs), annotations, and features. We then propose an extension of the SQL syntax and the query analyzer to support this new operator. We compared the two state-of-art algorithms against several SQL statements and found when to use each one of them in order to improve query time execution. This work incorporates and studies the behavior of several similarity-aware division algorithms in a commercial RDBMS. Recent works focus on extending relational operators to support similarity comparisons and their inclusion in relational database management systems. These data are better compared by similarity, whereas relational algebra always compares data by equality or inequality. However, the relational division is unable to support the needs of modern applications that manipulate complex data, such as images, audio, long texts, genetic sequences, etc. The division operator from the relational algebra allows simple and intuitive representation of queries with the concept of “for all”, and thus it is required in many real applications.
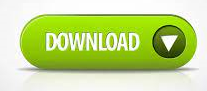